Data Lake Vs Data Warehouse: A Comprehensive Guide
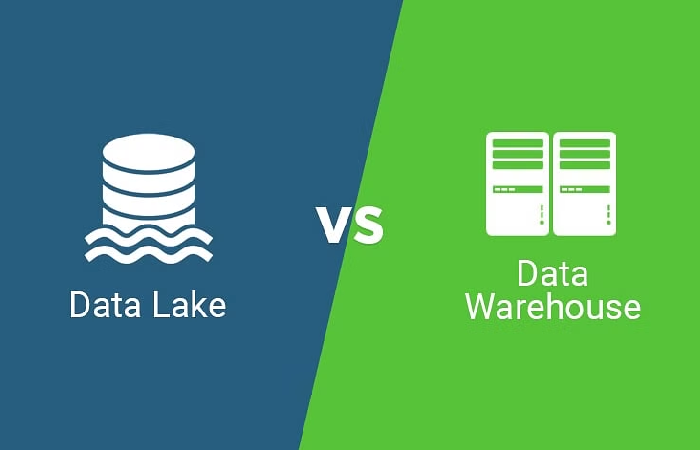
The development of data storage and analysis technologies has been one of the fields I have seen grow and change the most. Hence, today, we will be focusing on the two terminologies that are the basis of the modern, yet, already accepted and recognized data management strategy: data lakes and data warehouses. I will demonstrate the differences, strengths, and usage of these two techniques in this article so that you can make an informed decision about your data management strategy.
Understanding Data Lakes
A data lake is a storage facility that integrates all of the various structured and unstructured data from any of the organelles. It is developed to hold the raw data in its authentic file formats until the situation permits its implementation in the scheduled orders.
Key Features of Data Lakes:
-
Keep the information in its basic format
-
Handle multiple data patterns
-
Creativity and flexibility are the top two performance parameters
-
Reading the files via a read-on-search approach
-
Usually, data is lower in cost when the data volumes are larger
Understanding Data Warehouses
The data warehouse is an integrated storage facility that relies on the generic, highly-processed and organized file to serve its operating purpose. It is used for the faster querying and more manageable structured data analysis.
Key Features of Data Warehouses:
-
Store processed and formatted information.
-
Optimized for quicker querying and data analysis.
-
Schema-on-writing mechanism is employed.
-
Mostly applied in business intelligence and reporting.
-
In addition to that historical data support is included.
Advantages of Data Lakes
-
For the data lake, being able to accept and treat highly different and various types of data (including those of heterogeneous nature) without moving it special treatment are is one of its strength that I will mention here.
-
It is like being flexible when you want to store any data without any preprocessing.
-
Scalability is not a problem since their system can just be modified to include more and more data not needed at the start of the dataprocess.
-
Additional saving comes when dealing with bulk data but the savings are only on the storage side as more data can be stored using the same amount of memory.
-
Brain machines can predict problems and calculate new patterns of data with the data lake learning techniques provided.
-
One more profit idea that the data lake supposes allows for an Open to Connect (OTC) type of data discovery to be made on the raw data and thus, data will disclose itself to the questions unknown.
Advantages of Data Warehouses
-
Data warehouses were built to cater to the needs of structured, high-performance data users who often ask for business intelligence using stored data.
-
Queries are rapid; thus, you can get data in little time and also( query) is too fast to be handled live.
-
Data consistency will be improved where all the operations and data processes feeding into that Snowflake Data Warehouse will be written in a kind of governance that is approved by the data manager and he/she can verify that the same governorship rules their system.
-
Moving into the future, economies will have more power behind the ability to look at the project from many different angles and from a historical view or any other way they wish to perceive the entire project.
-
Moreover, data is cleaned for that problem solving ages and techniques.
-
Security becomes a priority and you now can deploy the latest technologies thus negate potential losses brought about by the increasing number of secured and non-secured users of your systems.
Comparing Data Lakes and Data Warehouses
To provide clearer explanations and help us understand very well the differences between data lakes and data warehouses, let us do the comparison to each of the subjects as shown below:
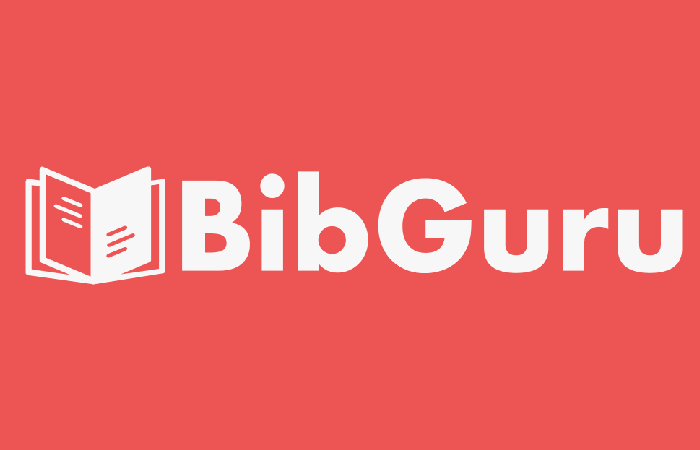
Use Cases and Industries
While they are used in modern-day data architecture, both data lakes and data warehouses are placed where it is right to put them. Here are some typical applications and industries that can benefit from both these technologies:
Data Lake Use Cases:
- Log analytics
- Machine learning model training
- Internet of Things (IoT) data processing
- Social media sentiment analysis
- Clickstream analysis
Industries benefiting from Data Lakes:
- Healthcare (for genomics research)
- Manufacturing (for sensor data analysis)
- Retail (for customer behavior analysis)
- Finance (for fraud detection)
- Energy (for smart grid optimization)
Data Warehouse Use Cases:
- Financial reporting
- Supply chain optimization
- Sales performance analysis
- Customer segmentation
- Marketing campaign analysis
Industries benefiting from Data Warehouses:
- Retail (for inventory management)
- Banking (for risk assessment)
- Insurance (for claims analysis)
- Telecommunications (for customer churn prediction)
- Healthcare (for patient outcomes analysis)
Current Trends and Future Developments
The cacophony of activities in data management has created the environment you and I live in and there are many trends that continue to shine thus we always see new developments in the data management area repeatedly. Here are the current trends and future developments that are currently underway in this field:
-
A singularity of data lakes and data warehouses (eg., Databricks' Lakehouse idea)
-
As cloud services technology continue to be customized, there will be a greater percentage of usage of cloud-based services.
-
The AI and machine learning will integrate well with other functionalities thus driving optimization of the applications and data manipulation supporting data governance and security features.
-
Better connection to data governors and secure data warehouse features will further decrease the threats of security breaches as the standardization and innovation continue.
-
The real-time simulation becomes higher as a result of new developments in hardware, software, and networks etc.
Choosing Between Data Lake and Data Warehouse
If you find yourself with such a decision to make as which between a "Data Lake" or "Data Warehouse" to use, what will be, preferably, the factors you will be putting in mind in such a situation? These can be identified as the following:
-
One of the great characteristics of the data lake is it acts as a no-schema repository that you can throw all kinds of data into.
-
For fast, structured queries, a data warehouse is good since it knows the location of the data where the query starts and stops.
-
Tech Heads that label the topic of Data Lake have their systems optimized to enhance raw data processing thus we can expect data lakes to beating out traditional Data Warehouse in potential data processing.
-
Query response by the computer can be like reading to the governor the following clean data of individuals that were sorted out after the highest their needs and that sort of control was reached because the government implemented strict guidelines and the best way the issue was dealt with was with people who safeguard the government from such as.
-
Such financial problems as the traditional data are certainly not new in the world and analytics helps to prevent such occurrences as developing and implementing new insights and methods like forecasting, planning, and budgeting and thus the cold chain companies can see the difference in the previous situations and be sure that there might be more informed decisions on their part.
-
Related to this ultimate question are the regulatory challenges and risks to losing data and damaging the integrity of the data lake, and/or data warehouses which are both applicable to this mainly but these could be the biggest arguments.
Conclusion
In my field, I have seen data lakes and data warehouses taking the essential place of the modern data architecture. As far as I am concerned, data lakes that are characterized by storing vast and unprocessed data and data warehouses that are focused on the query processing, are unequivocally some of the most important things to have for most organizations dealing with a huge amount of data.
Apart from the abstract knowledge you have, instead, the more specific knowledge gathered by evaluating your needs, the data types you have, and the types of analysis you are looking for, you are obliged to embark on the way of your organization's journey into digital space.
By getting to know the abilities and weaknesses of the data lakes and data warehouses, you are most likely to take the right steps to maximize your company's transformation through the optimal utilization of the data they carry.